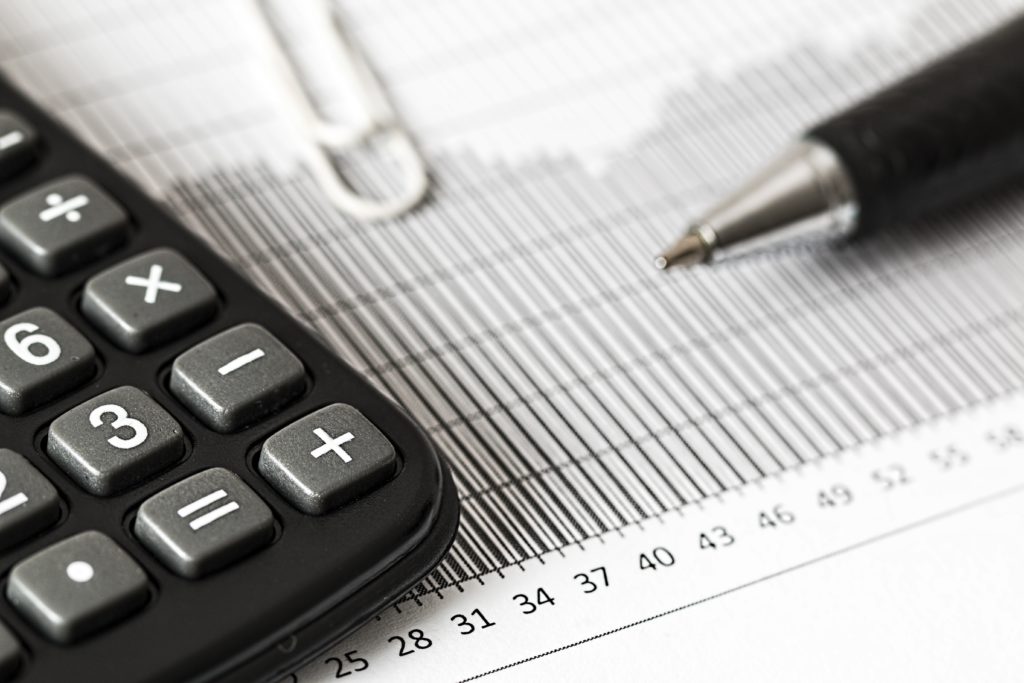
Fair lending analysis attempts to use lending transaction data to tell the story of the evenhandedness of underwriting, pricing, and marketing decisions in terms of what happened when, where, why, how, and to whom. Fair lending compliance managers may use HMDA data to quantify fair lending risk in several ways:
- Disparity Ratios. Comparing decision or outcome rates of one prohibited basis group to another to create disparity ratios.
- Statistically Significant Prohibited Basis Group Differences. Determining whether disparities between control and target groups are statistically significant, i.e., unlikely to have occurred by chance. If the differences are not significant, then we say that the outcome between the groups are not different in a statistical sense, although there may be a nominal observable difference.
- Transactional File Analysis. This involves a side-by-side comparison of control and target applicants or borrowers to determine whether applicants that were similarly situated in terms of applicant loan qualifications have a pattern of different outcomes.
- Multivariate Inferential Statistics. Using inferential statistics, like regression analysis, to control for differences in applicant qualifications and loan characteristics. This type of analysis looks to see whether the differences in outcomes are explained by legitimate risk-based factors rather than a statistically significant prohibited basis factor like race, ethnicity, sex, age or even some combination of the foregoing.
- Statistically Significant Differences in Market Lending Activity by Census Tract (AKA “Redlining”). Lenders are expected to take applications and make loans without regard to the racial or ethnic characteristics of the markets they serve. There is cause for concern when there are statistically significant market penetration differences in what are majority-majority census tracts and majority-minority tracts (Asian, Hispanic, Black, etc.). This can be even more complicated that other analyses because the data of your peers are involved in the equation.
There are many ways in which any single test, or all, of the analyses above can go wrong. As reported before, a lender may pass all the CFPB HMDA edit checks but still have HMDA data “inaccuracies.” These HMDA data inaccuracies may be manifested as missing applications or government monitoring information, illogical reporting, evidence of HMDA guideline misinterpretation, highly suspicious or outlier values, or just plain bad data. There is also the possibility that the edit checks are wrong or have been changed. ComplianceTech’s suite of products: HMDA Ready™, LendingPatterns™, and Fair Lending Magic™ are designed to help to identify HMDA data inaccuracies that are blind to the CFPB edit checks but could nevertheless result in compliance management risk for the lender.
Recent Comments